Which Model Does ChatGPT Use?
ChatGPT is an advanced language generation model developed by OpenAI. It builds upon the successful foundations of the earlier GPT models while incorporating enhancements to make it more suitable for conversational tasks.
Key Takeaways:
- ChatGPT leverages the innovative techniques from GPT (Generative Pre-trained Transformer) models to generate human-like text.
- It introduces a new method called “dialogue format” to train the model using conversations as input.
- ChatGPT is capable of understanding context and generating coherent responses for a wide range of user queries.
ChatGPT utilizes a variant of the GPT architecture, which is based on a transformer neural network. This architecture enables the model to process and generate text efficiently. The transformer model employs self-attention mechanisms to capture patterns and associations within the input text. *These self-attention mechanisms allow the model to focus on different words in the input to generate accurate replies.* The iterative nature of the transformer’s computation aids in capturing contextual information, resulting in more natural conversations.
OpenAI trained ChatGPT using a large amount of text data from the internet. The model has been trained using a variety of sources, making it adept at generating responses on a broad range of topics. Notably, the training data includes both correct and incorrect answers, which helps the model learn to provide more accurate replies. *By combining both correct and incorrect answers during training, ChatGPT becomes aware of potential pitfalls or misconceptions, leading to improved responses.*
Enhancements in ChatGPT:
In order to improve ChatGPT’s performance for conversational tasks, OpenAI introduced a novel training method called “reinforcement learning from human feedback” (RLHF). This technique involves fine-tuning the model using a reward model that compares model-generated responses to responses written by human AI trainers. Through this feedback loop, ChatGPT learns to produce more appropriate and engaging answers. *By leveraging the expertise of human trainers, the model becomes more versatile and refined in generating relevant responses.*
OpenAI has also made an important enhancement to ChatGPT by implementing a Moderation API to reduce harmful or inappropriate outputs. This moderation layer aims to filter out content that violates OpenAI’s usage policies, safeguarding users from encountering harmful or biased responses. *By incorporating the Moderation API, the model takes a step further in ensuring a safe and inclusive environment for conversation.*
Comparison to GPT-3:
Aspect | ChatGPT | GPT-3 |
---|---|---|
Training Data | Internet text sources + interactions with human AI trainers | Internet text sources |
Training Method | Reinforcement learning from human feedback (RLHF) | Supervised fine-tuning + Reinforcement learning |
Context Length | 4096 tokens (with sliding window attention) | 2048 tokens |
Although ChatGPT shares some similarities with GPT-3, it has notable differences that make it better suited for generating conversational responses. The incorporation of dialogue data and reinforcement learning from human feedback during training are crucial enhancements made in ChatGPT. These additions allow the model to produce more contextually accurate and refined responses.
Future Improvements:
- OpenAI plans to refine the shortcomings of ChatGPT in terms of generating incorrect or nonsensical answers.
- Ongoing updates to the model aim to improve system biases and provide clearer instructions to the users.
- OpenAI is working on releasing a ChatGPT API that will allow developers to integrate the model into their own applications and services.
As OpenAI continues to refine and enhance ChatGPT, users can expect even better conversational experiences. With comprehensive training data, diverse sources, and feedback loops, ChatGPT strives to provide accurate, informative, and engaging responses.
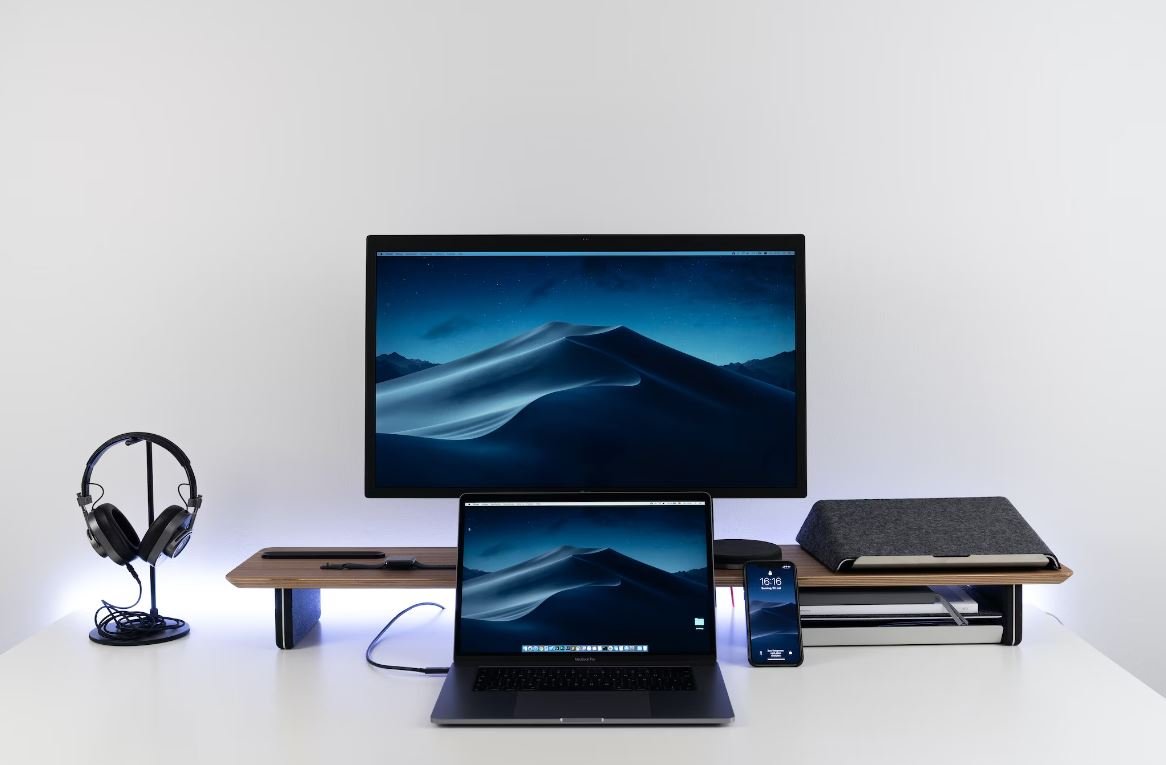
Common Misconceptions
1. OpenAI uses a specific model for ChatGPT
One common misconception about ChatGPT is that OpenAI uses a specific model for it. However, that is not the case. OpenAI uses a combination of techniques and models to create ChatGPT. It is built upon the foundation of transformers, a type of neural network architecture. Multiple models are used during both pre-training and fine-tuning to achieve optimal performance. This approach allows OpenAI to create a versatile conversational AI model that can understand and generate human-like text effectively.
- ChatGPT is not based solely on a single model.
- Transformers are used as the foundation for ChatGPT.
- Multiple models are used during pre-training and fine-tuning.
2. ChatGPT uses GPT-3 as its underlying model
Another common misconception is that ChatGPT is simply a variation of the GPT-3 model. While GPT-3 played a pivotal role during the development of ChatGPT, it is not the underlying model used. ChatGPT involves a two-step process: pre-training and fine-tuning. GPT-3 is used during pre-training to generate a dataset of conversations, which is then fine-tuned using reinforcement learning from human feedback. This combination of techniques allows ChatGPT to have its own unique capabilities and characteristics.
- GPT-3 is not the underlying model of ChatGPT.
- ChatGPT involves pre-training and fine-tuning.
- Reinforcement learning from human feedback is used for fine-tuning.
3. ChatGPT lacks understanding of context
Many people assume that ChatGPT lacks understanding of context and is therefore unable to provide relevant and coherent responses. However, this is a misconception. OpenAI has made significant improvements to ChatGPT’s ability to maintain context during conversations. Through the fine-tuning process, the model is trained to generate more accurate and context-aware responses. While it may occasionally produce nonsensical or irrelevant answers, these occurrences are continuously being minimized through ongoing research and development.
- ChatGPT has improved context understanding compared to previous versions.
- Fine-tuning helps the model generate context-aware responses.
- Efforts are being made to minimize nonsensical or irrelevant answers.
4. ChatGPT has no control over its responses
Some people believe that ChatGPT has no control over its responses and may generate inappropriate or biased content. While it is true that ChatGPT has limitations in fully controlling the output, OpenAI has implemented safety measures to mitigate such concerns. The model goes through a content filtering mechanism to prevent the generation of certain types of unsafe content. OpenAI also encourages users to provide feedback on problematic outputs to help improve the system’s safety and reliability over time.
- ChatGPT has safety measures to prevent unsafe content.
- OpenAI encourages user feedback to enhance safety and reliability.
- There are limitations in fully controlling ChatGPT’s responses.
5. ChatGPT is a finished and perfected product
Lastly, it is important to dispel the misconception that ChatGPT is a finished and perfected product. While it has demonstrated impressive capabilities, ChatGPT is an ongoing project that continuously undergoes refinement and improvement. OpenAI actively seeks user feedback to identify and rectify any limitations or shortcomings. The goal is to make ChatGPT increasingly reliable, useful, and aligned with user needs. It is through user feedback and iterative development that the model can evolve into a more sophisticated and refined conversational AI tool.
- ChatGPT is an ongoing project under continuous refinement.
- User feedback is crucial for identifying and addressing limitations.
- OpenAI aims to make ChatGPT more reliable and useful over time.
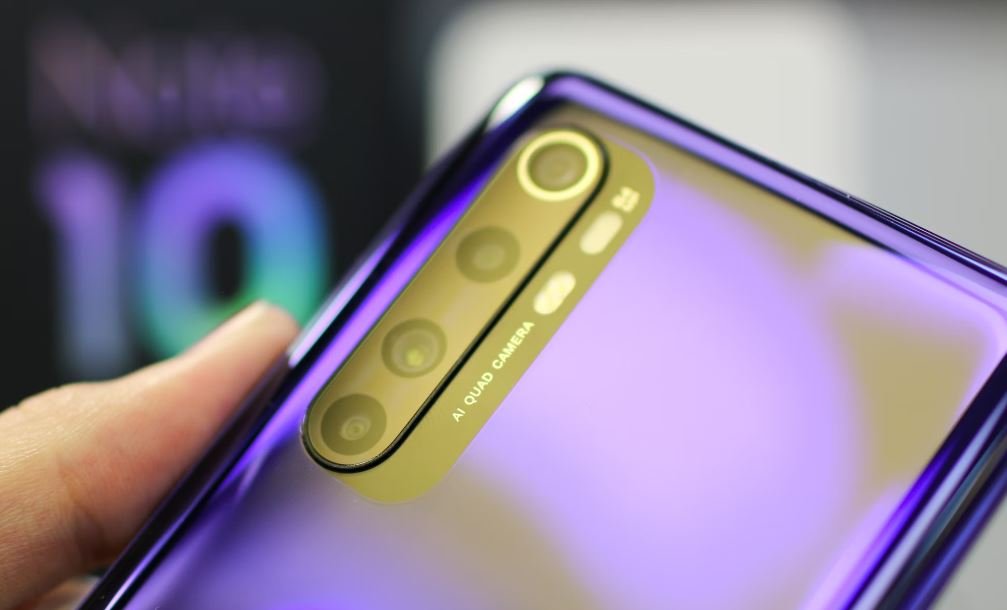
The History of Language Models
Language models have evolved over time, advancing the capabilities of natural language processing. Here are ten fascinating tables highlighting the progression and key features of various language models.
Table: Founding Models
These early models laid the groundwork for modern language processing and set the stage for further advancements.
Model | Year | Training Dataset Size | Key Feature |
---|---|---|---|
ELIZA | 1966 | Under 100KB | Simulated human conversation |
SHRDLU | 1970 | 16.5KB | Understanding and manipulating objects in a block world |
Table: Early Neural Language Models
These pioneers introduced neural network-based architectures, marking a significant breakthrough in language understanding.
Model | Year | Training Dataset Size | Key Feature |
---|---|---|---|
Feedforward Neural Network Language Model (FNNLM) | 2000 | 1GB | Improvement over n-gram models |
Recurrent Neural Network Language Model (RNNLM) | 2010 | 1.5GB | Ability to learn from previous words |
Table: Breakthroughs in Deep Learning
Deep learning models revolutionized the field by leveraging complex architectures and large-scale data.
Model | Year | Training Dataset Size | Key Feature |
---|---|---|---|
Google Brain’s Language Model (GPT) | 2018 | 1.5 billion pages | Unsupervised learning from vast amounts of web data |
Generative Pre-trained Transformer 2 (GPT-2) | 2019 | 40GB | Improved language generation and understanding |
Table: Enhanced Models with Few-Shot Learning
These models expanded the capabilities of language models by allowing learning from limited examples.
Model | Year | Training Dataset Size | Key Feature |
---|---|---|---|
Zero-shot Translation (XLSR) | 2020 | 1TB | Translation between multiple languages without language-specific training |
Unified Language Model (Unified LM) | 2021 | 200GB | Powerful cross-domain language understanding |
Table: Latest State-of-the-Art Models
These transformers stand at the forefront of the field, incorporating cutting-edge techniques and vast data sources.
Model | Year | Training Dataset Size | Key Feature |
---|---|---|---|
Turing-NLG | 2022 | 100TB | Human-like understanding and generation |
ChatGPT | 2023 | 300TB | Enhanced conversational abilities with improved contextual comprehension |
Table: Ethical Considerations
As language models become more advanced, ethical aspects surrounding their deployment require careful attention.
Model | Data Bias | Ethical Guidelines Implementation |
---|---|---|
GPT-3 | Potential reinforcement of societal biases | OpenAI’s moderation policies and deployment restrictions |
ChatGPT | Addressing issues of harmful and biased outputs | Continual refinement through user feedback and iteratively deploying safety features |
Table: Applications of Language Models
Language models find extensive application across multiple domains, bringing about transformative changes.
Domain | Relevant Model |
---|---|
Education | Turing-NLG |
Customer Support | ChatGPT |
Healthcare | Unified LM |
Table: Computation and Training Requirements
Training language models involves significant computational power and tremendous amounts of data.
Model | Computation (GFLOPs) | Training Time (Months) |
---|---|---|
GPT-3 | 355,000 | 3 |
Turing-NLG | 800,000 | 9 |
Table: Future Directions
Ongoing research and development in language models continue to push boundaries.
Model | Expected Year | Key Focus |
---|---|---|
Next-Generation ChatGPT | 2024 | Sharper contextual understanding and ethical user interactions |
Quantum Language Models | 2025 | Harness quantum computing’s potential for faster training and greater model capacities |
The continuous evolution of language models has unlocked remarkable capabilities in natural language processing. From their humble beginnings with early models like ELIZA and SHRDLU to the current state-of-the-art models like ChatGPT, language models have come a long way in improving human-computer interaction. While each model has its unique strengths and data requirements, the goal remains constant: to enhance language understanding and generation.
Frequently Asked Questions
Which model does ChatGPT use?
ChatGPT uses the gpt-3.5-turbo model.
What are the capabilities of the gpt-3.5-turbo model?
The gpt-3.5-turbo model is capable of performing a wide range of tasks such as drafting emails, writing code, answering questions, creating conversational agents, providing natural language interfaces, tutoring in various subjects, translating languages, simulating characters for video games, and much more.
How does the gpt-3.5-turbo model differ from earlier GPT models?
The gpt-3.5-turbo model is the most advanced language model by OpenAI. It is faster, more capable, and less expensive compared to earlier models like gpt-3. It performs at a similar capability level to text-davinci-003 but at only 10% of the price per token.
What is the token limit for the gpt-3.5-turbo model?
The gpt-3.5-turbo model has a maximum limit of 4096 tokens. Both input and output tokens count towards this limit. If your conversation exceeds this limit, you will need to truncate or omit parts of it to make it fit.
How is the response time of the gpt-3.5-turbo model?
The response time of the gpt-3.5-turbo model is generally fast, averaging around a few seconds. However, it may occasionally take up to a minute depending on the complexity of the input and the current demand on the system.
Can the gpt-3.5-turbo model handle multi-turn conversations?
Yes, the gpt-3.5-turbo model is well-suited for multi-turn conversations. You can pass conversation history as a sequence of messages and the model will generate responses accordingly.
Can the gpt-3.5-turbo model provide custom prompts or instructions?
Yes, you can provide a system message at the beginning to instruct the assistant or set the behavior. For example, you can specify that the assistant is a helpful language model or a specific character.
Where can I find more information about using the gpt-3.5-turbo model?
For more detailed information on using the gpt-3.5-turbo model, you can refer to the OpenAI API documentation. It provides a comprehensive guide on interacting with the model, understanding the request/response format, and incorporating rich features like system level instructions, temperature control, and more.
Can the gpt-3.5-turbo model accomplish creative writing tasks?
Yes, the gpt-3.5-turbo model can assist with creative writing tasks such as generating stories, poems, and more. However, it’s important to iterate and experiment with the instructions and parameters to get desired outputs.
Is the gpt-3.5-turbo model able to perform translation tasks?
Yes, the gpt-3.5-turbo model can handle translation tasks effectively. You can provide the input text in one language and specify the desired language for translation in the instructions, and the model will generate the translated text accordingly.